Hi,
I'm trying to try DeepLearningSuite, but I don't know if I'm doing it the right way. I am able to run DatasetEvaluationApp, but I get a warning that I don't know if it should come out:
datasetPath
/sampleFiles/datasets/home
evaluationsPath
/sampleFiles/evaluations
inferencesPath
/sampleFiles/evaluations
namesPath
/sampleFiles/cfg/SampleGenerator
netCfgPath
/sampleFiles/cfg/darknet
weightsPath
/sampleFiles/weights/yolo_2017_07
datasetPath
/sampleFiles/datasets/home
evaluationsPath
/sampleFiles/evaluations
inferencesPath
/sampleFiles/evaluations
namesPath
/sampleFiles/cfg/SampleGenerator
netCfgPath
/sampleFiles/cfg/darknet
weightsPath
/sampleFiles/weights/yolo_2017_07
WARNING: Logging before InitGoogleLogging() is written to STDERR
W0129 17:31:21.528326 14332 ListViewConfig.cpp:89] path: /sampleFiles/weights/yolo_2017_07 does not exist
W0129 17:31:21.528415 14332 ListViewConfig.cpp:89] path: /sampleFiles/cfg/darknet does not exist
W0129 17:31:21.528518 14332 ListViewConfig.cpp:89] path: /sampleFiles/cfg/SampleGenerator does not exist
The result is:
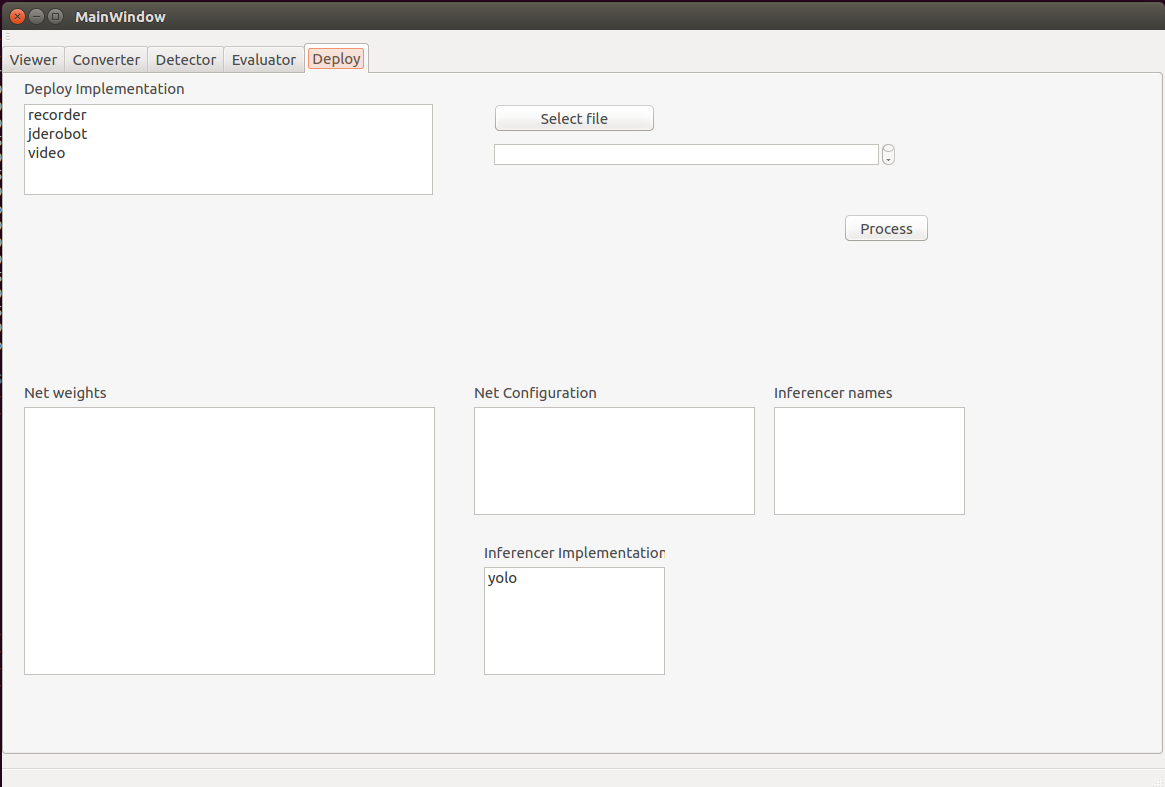
My appConfig.txt is:
--datasetPath
/mnt/large/pentalo/deep/datasets
--evaluationsPath
/mnt/large/pentalo/deep/evaluations
--weightsPath
/mnt/large/pentalo/deep/weights
--netCfgPath
/mnt/large/pentalo/deep/cfg/darknet
--namesPath
/mnt/large/pentalo/deep/cfg/SampleGenerator
--inferencesPath
/mnt/large/pentalo/deep/evaluations
Also, I tried to run SampleGenerationApp and the result is:
WARNING: Logging before InitGoogleLogging() is written to STDERR
W0129 17:25:17.989045 13853 SampleGenerationApp.cpp:99] Key: outputPath is not defined in the configuration file
W0129 17:25:17.989125 13853 SampleGenerationApp.cpp:99] Key: reader is not defined in the configuration file
W0129 17:25:17.989130 13853 SampleGenerationApp.cpp:99] Key: detector is not defined in the configuration file
The configuration file is:
--outputPath
/URJC
--dataPath
/sampleFiles/images
--detector
#datasetReader
deepLearning
#pentalo-bg
--inferencerImplementation
yolo
--inferencerNames
/sampleFiles/cfg/SampleGenerator/person1class.names
--inferencerConfig
/sampleFiles/cfg/darknet/yolo-voc-07-2017.cfg
--inferencerWeights
/sampleFiles/weights/yolo_2017_07/yolo-voc-07-2017.weights
--reader
#spinello
recorder-rgbd
--readerNames
none
Thank you very much,
Regards
.